Data science and product management often feel like separate roles—one focused on building models, the other on shaping business strategy. But more data scientists are stepping into product management, realizing that their insights can do more than optimize models—they can shape entire products.
Claire Corthell, a former data scientist turned product manager at Lyft, explains why this transition is happening. Many companies struggle to turn data insights into real business impact, and product teams often overlook the full potential of data-driven decision-making. The result? A disconnect that slows progress.
The key to bridging this gap is a mindset shift. Instead of just asking, “What can the data tell us?”, aspiring product managers must start asking, “What problem are we solving?” This article explores why data scientists are making the shift, the challenges they face, and a step-by-step guide to successfully transitioning into product management.
Understanding the Relationship Between Data Science and Product Management
Data science and product management serve different functions but should work together. Product managers define problems and prioritize solutions, while data scientists provide insights to guide decisions. When aligned, they create data-driven products that serve users better. However, when disconnected, valuable insights go unused, and decisions lack a solid foundation.
The Role of a Product Manager in a Data-Driven Company
A product manager’s job is to define problems, prioritize solutions, and align teams. They don’t need to be data scientists, but they must know how to apply data insights to make better product decisions.
Clare Corthell explains that many companies struggle to integrate data effectively. Teams build models, but without a clear product vision, those insights don’t drive real impact. A good PM bridges this gap, ensuring data leads to meaningful improvements.
Without this alignment, data remains a side project instead of shaping key product decisions. Product managers who understand data can ask better questions, interpret results, and make informed choices that benefit both the business and the user.
How Data Science Fits into Product Strategy
Data science enhances products, improves user experience, and optimizes decision-making. Companies rely on data for predicting demand, personalizing experiences, and improving efficiency.
Take Lyft: every ride request involves ETA predictions, optimal routes, and driver assignments—all powered by data. Initially, their system relied on simple straight-line estimates, but with data-driven improvements, pickups became faster and more accurate.
However, data science is only valuable if applied correctly. Experimentation, A/B testing, and predictive models mean nothing unless they translate into real product improvements. Product managers play a key role in ensuring data doesn’t just stay in reports but drives action.
The Gap Between Data Science and Product Teams
Despite working toward the same goal, product managers and data scientists often struggle to align. PMs think in terms of user needs and business goals, while data scientists focus on models and accuracy. This misalignment leads to missed opportunities.
One major issue is communication—data teams speak in probabilities, while PMs need clear insights. Another challenge is timelines—PMs work on tight schedules, while data projects often require deep research. When priorities clash, data science becomes reactive instead of proactive.
The solution? Collaboration from the start. Clare emphasizes that data scientists should be involved early in the product process, not just when an analysis is needed. Clearer communication, shared goals, and a better understanding of each role can turn data into a real driver of product success.
Clare’s Path from Consulting to Product Management
Before stepping into product management, Clare ran a data science consultancy, working with companies worldwide. She quickly realized a common pattern—businesses were excited about data science but struggled to apply it effectively. Organizations lacked the structure to integrate insights into real product decisions, leaving valuable data unused.
Seeing this gap firsthand, she transitioned into product management to help bridge the disconnect between data teams and decision-makers. Her experience taught her that having the right data isn’t enough—companies need the right people to translate insights into action. This shift from consulting to product helped her leverage her data expertise in a more strategic way, ensuring that insights drove real business impact.
Why Data Scientists Are Transitioning into Product Management
More data scientists are stepping into product management, realizing their work can influence more than just models—it can shape business strategy. The shift isn’t just about career growth; it’s about impact. Data scientists often hit roadblocks when their insights don’t translate into real decisions. Product management offers a way to drive change, not just analyze it.
The Limitations of a Pure Data Science Role
Many data scientists feel disconnected from real business impact. They build models, run experiments, and generate insights, yet they often see their work ignored or underutilized.
Clare Corthell highlights a common issue—companies invest in data science but struggle to implement its findings effectively. Models get built, but teams don’t always know how to apply them to product decisions. Even when insights are valuable, business priorities may shift, leaving data projects sidelined.
Another challenge is deployment. A model isn’t useful unless it integrates smoothly into a product. However, many companies lack the infrastructure or alignment to make this happen. The result? Data scientists create solutions, but users never see the benefits.
As businesses rely more on data-driven decisions, there’s a growing demand for professionals who can connect technical insights to business strategy. This is where product management comes in.
The Benefits of Moving to Product Management
Transitioning to product management expands influence beyond technical execution. Instead of just analyzing problems, product managers define which problems to solve and how to solve them.
Clare points out that product managers have the power to drive decisions, prioritize projects, and ensure that data insights turn into real product improvements. This shift allows former data scientists to take an active role in shaping user experiences, not just optimizing them after the fact.
Another major benefit is the cross-functional impact. Product managers work closely with engineers, designers, and business teams, making decisions that balance user needs, technical feasibility, and business goals. This broadens career growth and opens doors to leadership roles.
Key Skills Data Scientists Already Possess That Help in Product Management
Data scientists already have several skills that make them strong product managers:
- Analytical and problem-solving mindset – Product managers need to make data-driven decisions. Data scientists excel at breaking down problems and identifying trends.
- Understanding of experimentation and metrics – Product managers rely on A/B testing, user analytics, and key performance indicators (KPIs). Data scientists are already skilled in these areas.
- Experience working with cross-functional teams – Data scientists often collaborate with engineers and business teams. This experience translates well into managing product development.
Now, here comes the good part: how can data scientists successfully transition into product management? Let’s break it down step by step.
The Core Challenges of Transitioning from Data Science to Product Management
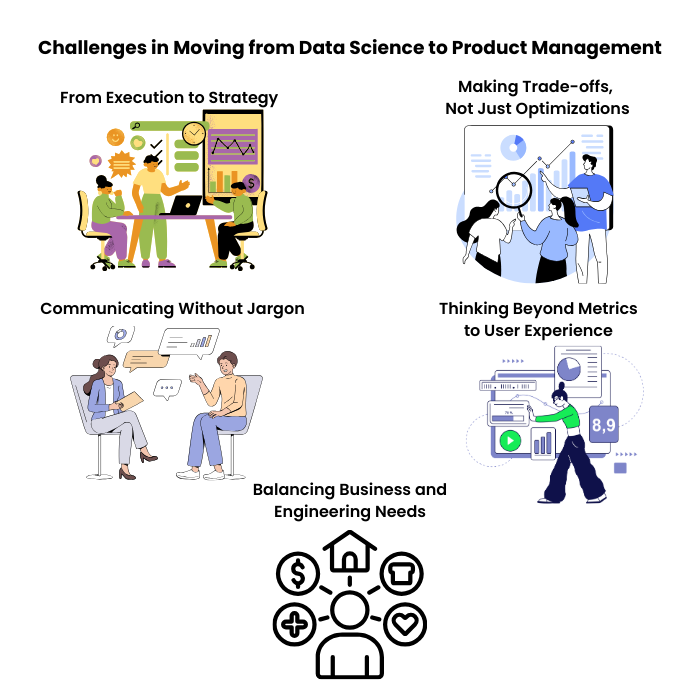
Moving from data science to product management isn’t just about learning business skills—it’s about changing how you think. Instead of refining models, you start defining problems and prioritizing what to build. This shift comes with challenges, but recognizing them early makes the transition smoother.
- From Execution to Strategy
Data scientists focus on solving problems with data, but product managers decide which problems matter most. Instead of optimizing a model, you’ll ask, “Does this even solve a real user need?” Clare Corthell explains that many companies struggle, not because they lack data, but because they don’t apply it effectively.
- Communicating Without Jargon
A great model is useless if no one understands its value. Executives, engineers, and designers don’t think in probabilities—they need clear, real-world impact. Instead of saying, “We improved accuracy by 12%,” a PM would say, “Riders now wait 30 seconds less, reducing cancellations.” Product managers must turn complex insights into clear, actionable takeaways.
- Making Trade-offs, Not Just Optimizations
In data science, the goal is accuracy. In product management, the goal is impact with limited resources. A perfect solution that takes a year to build might not be as useful as a simple fix that solves 80% of the problem today. PMs balance effort vs. impact rather than always chasing the most precise solution.
- Thinking Beyond Metrics to User Experience
Data scientists focus on precision, recall, and statistical confidence. Product managers think in terms of user engagement, retention, and revenue. It’s not just about numbers—it’s about whether the product works better for customers.
- Balancing Business and Engineering Needs
Just because something is possible doesn’t mean it’s worth building. PMs must weigh technical feasibility against business value. A complex machine learning model might improve recommendations, but if a simple rules-based system works 90% as well, it may be the smarter choice.
The Evolving Relationship Between Data Science and Product Management
Product management and data science are no longer separate fields—they are increasingly connected. Companies rely on data-driven decisions more than ever, making it essential for product managers to understand data and for data scientists to think beyond models. But what does the future hold? Will PMs become more technical, or will data scientists take on business roles?
Will Product Managers Need to Become More Technical?
Clare Corthell believes that future product managers will need a strong grasp of experimentation, analytics, and metrics. It’s no longer enough to rely on gut instinct—business decisions are now backed by data.
Understanding A/B testing, SQL, and key performance metrics helps PMs work more effectively with data teams. They don’t need to build machine learning models, but they should know what’s possible and how to use data to drive decisions.
Think of it like a carpenter with a toolbox. You don’t need to be an expert in every tool, but you should know which one to use and when. The same applies to product managers—they don’t need to code, but they should understand how data works to make smarter decisions.
Will Data Scientists Need to Become More Business-Oriented?
On the other side, data scientists are expected to think beyond models and consider business impact. It’s not just about predicting outcomes—it’s about asking, “How does this help the user? How does this improve revenue?”
Many data teams struggle to communicate their value to the business. Clare explains that product managers don’t always understand what data scientists can offer, and data scientists don’t always frame their insights in a way that influences product decisions. The best data scientists know how to tell a compelling story with data, linking their work to clear business results.
As companies integrate data science deeper into decision-making, data professionals who understand business strategy will have a major advantage. The more they can connect insights to action, the more valuable they become.
How Can Insights from Data Strategy and Governance Aid in Transitioning from Data Science to Product Management?
Incorporating data strategy insights into product management enables a seamless transition from data science. By aligning product goals with data-driven decisions, teams can enhance user experiences and drive innovation. These insights foster collaboration between data scientists and product managers, ensuring products meet market needs effectively and efficiently.
How to Successfully Transition from Data Science to Product Management
Moving from data science to product management isn’t just about switching roles—it’s about changing your approach. Instead of focusing on data accuracy and model performance, you’ll start thinking about business impact and user needs. Clare Corthell shared insights on what makes this transition successful. Here’s a step-by-step guide to help you make the shift.
Step 1: Understand the Core Responsibilities of a Product Manager
Product management is about identifying the right problems to solve and making sure the solutions align with business goals and user needs. Unlike data science, where you analyze existing data, PMs focus on defining what needs to be built and why.
Start by studying how product decisions are made. Read product management books, follow industry leaders, and observe how PMs in your company prioritize features. Ask yourself:
- What drives a product’s success?
- How do companies decide what to build next?
- How do product managers balance user experience, business impact, and technical feasibility?
So read on, because the next step is where you start applying what you have learned.
Step 2: Improve Communication and Storytelling
Data scientists often present findings in technical terms, but product managers must translate insights into clear, actionable business decisions. A model with 98% accuracy might be impressive, but an executive cares more about how it improves user experience or revenue.
Practice framing insights as stories. Instead of saying, “Our churn prediction model is 85% accurate,” say, “With this model, we can identify at-risk customers early and offer discounts to retain them.” The better you explain impact, the easier it is to influence decisions.
Clare emphasizes that successful PMs simplify complex ideas without losing meaning. Watch how experienced PMs communicate—how they frame problems, present trade-offs, and justify decisions.
Step 3: Gain Hands-On Product Experience
You don’t need a product manager title to start thinking like one. Look for opportunities in your current role to take on product-related tasks.
- Work closely with product managers and ask to sit in on roadmap discussions.
- Take ownership of projects that involve defining business problems, not just analyzing data.
- Helped design A/B tests and translate findings into actionable recommendations.
Clare highlights that many data scientists already contribute to product decisions without realizing it. Shifting from an execution mindset to a decision-making one is the key.
Step 4: Learn Business Fundamentals
Data scientists are great at measuring things, but understanding why those metrics matter is what makes a great product manager. PMs think in terms of growth, engagement, and revenue.
Learn the basics of:
- How companies make money (subscriptions, transactions, ads).
- What metrics businesses care about (customer lifetime value, churn rate, conversion rates).
- How product decisions impact the bottom line.
Clare points out that many PMs come from business backgrounds, not technical ones. A data scientist who understands business has a huge advantage.
Step 5: Make the Move—Apply or Transition Internally
Once you’ve built product knowledge and gained hands-on experience, it’s time to step into a PM role. You can:
- Apply for Associate Product Manager (APM) or Technical PM roles.
- Use your data expertise as a selling point—companies love PMs who understand analytics.
- Transition within your company—many companies prefer to promote internally.
Clare’s journey from data science to product wasn’t overnight, but she made it by gradually taking on more product responsibilities until it became a natural transition. If you start applying these steps today, you’ll be on the same path.
Conclusion: Turning Insights Into Action
Transitioning from data science to product management isn’t just about a new role—it’s about thinking differently. Instead of focusing only on models and accuracy, you start asking bigger questions: What problem are we solving? How does this improve the product?
Clare Corthell’s journey highlights a key lesson: Data is only valuable if it drives action. Too often, insights get stuck in reports instead of shaping real decisions. That’s why more data scientists are stepping into product roles—to ensure their work creates real impact.
If you’re looking to make the shift, start small—own business-driven projects, sharpen your communication, and learn how products are built. The demand for data-driven product managers is growing, and if you can bridge the gap between data and decisions, you’ll be the one shaping what comes next.