Data is the backbone of every modern business, but having data isn’t enough. The real challenge is turning it into a strategic asset that drives growth, innovation, and compliance. Yet, many organizations struggle with scattered data, poor governance, and a lack of direction. Without a clear plan, companies waste time answering basic questions, make decisions on insufficient data, and risk falling behind competitors who get it right.
Greg Hahn, a data strategy leader with deep experience in governance, shares a simple truth—companies must slow down to speed up. Rushing into AI, automation, and analytics without strong data foundations leads to inefficiency and failure. Instead, organizations need a structured approach: define a data strategy, establish governance, and align data efforts with business goals.
In this discussion, Greg breaks down the data strategy maturity curve, explains why many companies operate in silos, and reveals how businesses can assess and improve their data management. He also dives into AI readiness, highlighting why most organizations aren’t prepared to take full advantage of artificial intelligence despite the hype.
So, if you’re wondering why your data initiatives aren’t delivering the expected results or how to build a strategy that works, keep reading. This article unpacks Greg’s insights and provides a roadmap for making data governance a competitive advantage.
What is Data Strategy and Why Does It Matter?
A data strategy is a roadmap for managing and using data effectively. Without it, businesses operate reactively, wasting time and resources trying to make sense of scattered information. A strong data strategy aligns with business goals, ensuring that data isn’t just collected but actively drives decisions and innovation.
Greg Hahn emphasizes that many organizations fail because they focus on data projects in isolation instead of having a company-wide approach. They treat data management as an IT function rather than a strategic asset. This siloed thinking limits impact, leading to poor data quality, governance gaps, and inconsistent decision-making.
Assessing Data Maturity: Where Does Your Company Stand?
Not all companies are at the same stage of data maturity. Some are just getting started, while others have advanced analytics and AI capabilities. However, growth isn’t always linear—companies often move forward in some areas while lagging in others.
Greg recommends a practical approach: assess data maturity based on immediate business needs, not five-year projections. The most effective strategies focus on the next year, adapting as the business evolves. Large corporations, despite having extensive data teams, often struggle due to outdated processes and bureaucratic hurdles.
To measure maturity, Greg suggests using a simple scale:
- Level 1: Data exists but is managed at a project level.
- Level 2: Data is structured within specific departments (finance, HR, marketing).
- Level 3: Data is governed across the enterprise.
- Level 4: Advanced analytics and AI are fully integrated.
- Level 5: The company leads the industry in data-driven decision-making (rare and expensive to maintain).
Most companies fall between Levels 2 and 3, meaning they have some structure but lack enterprise-wide data governance. Using proven frameworks can help businesses quickly assess their current state and identify areas for improvement without reinventing the wheel.
So, if your organization struggles with messy data, unclear ownership, or slow decision-making, the first step isn’t AI—it’s building a solid data foundation.
The Critical Role of Governance in Data Strategy
Why Governance is the Foundation of Data Success
Data governance isn’t just about compliance—it’s the foundation for making data useful, scalable, and efficient. Without it, organizations spend more time fixing data issues than leveraging it for strategic decisions. Poor governance leads to duplicate records, inconsistent reporting, and unreliable insights, ultimately slowing down business growth.
Greg Hahn emphasizes the idea of “slow down to speed up.” Many companies rush into AI and analytics without first ensuring data quality and governance. This results in flawed models and wasted investments. Establishing governance upfront ensures data is accurate, accessible, and aligned with business needs, allowing organizations to scale without running into bottlenecks.
Common governance mistakes include:
- Treating governance as an IT-only responsibility instead of a company-wide initiative.
- Focusing on short-term fixes rather than sustainable data management.
- Neglecting data ownership, leading to confusion over accountability.
How Companies Can Implement Effective Data Governance
Governance shouldn’t be a roadblock—it should enable smarter, faster decision-making across the organization. Breaking down silos is key. Instead of each department managing data in isolation, a unified governance approach ensures consistency and trust in the data being used.
Greg’s team implemented a governance framework to assess a large bank’s data capabilities. Using a streamlined maturity assessment, they quickly identified gaps and structured a plan to improve data management without overwhelming the organization. The key was focusing on practical steps: assigning data owners, setting clear quality standards, and integrating governance into everyday workflows.
Good governance doesn’t just prevent problems—it creates opportunities. When businesses manage data effectively, they can innovate faster, reduce risk, and gain a competitive edge. So, before jumping into advanced analytics, organizations should ask themselves: Is our data ready to support real growth?
The Connection Between Data Governance and AI Readiness
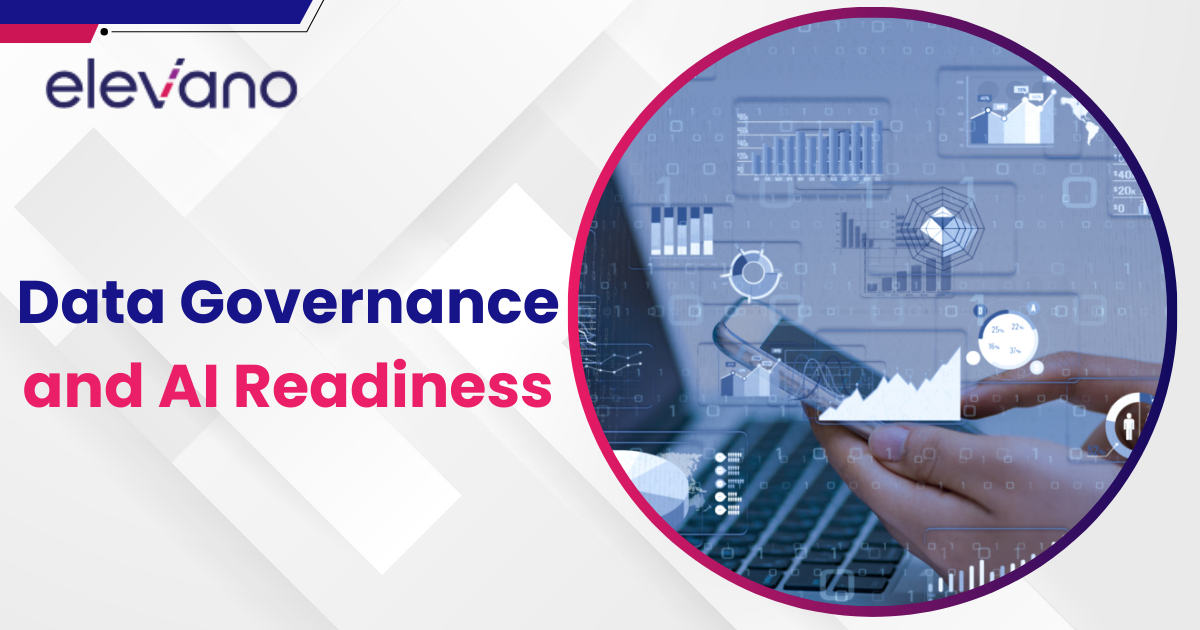
Why AI is Only as Good as the Data Behind It
AI is only as reliable as the data feeding it. Without strong governance, companies risk training AI models on incomplete, inaccurate, or biased data, leading to flawed predictions and costly mistakes. Many organizations rush to adopt AI without addressing foundational data challenges, setting themselves up for failure.
Greg Hahn has spoken with over 50 companies about AI adoption, and most share a common issue: They’re eager to implement AI, but their data isn’t ready. Poor governance, inconsistent quality controls, and a lack of alignment between business and data teams create major gaps in AI readiness. If companies don’t fix these issues first, AI initiatives end up delivering unreliable insights or, worse, damaging business outcomes.
The Five Key Pillars of an AI-Ready Data Strategy
For AI to deliver real value, companies must ensure their data strategy is solid before adoption. Greg highlights five critical pillars that determine AI readiness:
- Business Alignment – AI should solve real business problems. Without a clear strategy, companies waste resources on hyped-up technology that doesn’t drive results.
- Data Literacy Across Teams – AI isn’t just for engineers. Every team—marketing, finance, HR—must understand and trust AI-driven insights. Without company-wide data literacy, AI adoption stalls.
- Accessible & Reliable Data – AI needs clean, structured, and easily retrievable data. Companies without a centralized data marketplace struggle with inefficiencies that slow AI implementation.
- Security & Compliance – Poor governance leads to biased AI models, privacy risks, and compliance failures. Strong data security and ethical AI policies must be in place.
- Scalable Infrastructure – Many companies still rely on outdated systems that weren’t built for AI. Cloud-based, flexible architectures enable real-time data access and future AI expansion.
Instead of rushing into AI, businesses must fix their data foundations first. AI doesn’t solve bad data—it magnifies existing issues. The smartest companies will focus on governance, quality, and security before expecting AI to drive results.
How Privacy Laws and Regulations are Shaping Data Governance
The Rising Importance of Data Privacy Compliance
Data privacy laws are becoming more stringent, and businesses can no longer afford to treat compliance as an afterthought. Regulations like GDPR (Europe), CCPA (California), and the proposed American Privacy Rights Act are putting increasing pressure on organizations to manage data responsibly. These laws don’t just affect consumer data—they directly impact how companies structure data governance, store information, and use AI.
AI relies heavily on vast amounts of data, but if companies don’t have clear governance frameworks in place, they risk violating privacy laws. Many organizations are already facing challenges in balancing AI innovation with privacy regulations. Without proper controls, AI models may process sensitive data without consent, leading to legal issues and loss of consumer trust.
To prepare for evolving privacy laws, businesses must strengthen governance policies by:
- Implementing clear data access and security measures.
- Creating transparent consent management processes.
- Regularly auditing AI models to prevent unintentional data misuse.
- Aligning privacy policies with regulatory frameworks to ensure compliance.
Instead of waiting for enforcement actions, companies should take a proactive approach to privacy, integrating compliance into their governance strategy from the start.
The Business Benefits of Proactive Privacy Management
Privacy compliance isn’t just about avoiding fines—it’s a competitive advantage. Companies that prioritize data privacy build stronger relationships with customers, avoid costly regulatory penalties, and improve internal efficiency.
Here’s how proactive privacy management benefits businesses:
- Stronger Customer Trust – Consumers are becoming more aware of data privacy risks. Companies that demonstrate transparency and ethical data use gain a trust advantage over competitors.
- Better Data Quality for AI – Privacy regulations force companies to refine their data collection and management processes. This results in cleaner, higher-quality data, which directly improves AI performance and reduces the risk of biased or flawed outcomes.
- Regulatory Risk Avoidance – Non-compliance can be expensive. Fines under GDPR can reach millions of dollars, and failing to protect consumer data can lead to reputation damage, lawsuits, and loss of business opportunities.
- Operational Efficiency – Companies with structured data governance processes spend less time fixing compliance issues and more time using data effectively. When privacy management is built into governance, businesses can scale AI and analytics initiatives with confidence.
Privacy regulations are only going to get stricter. Instead of viewing compliance as a burden, companies should see it as an opportunity to create a more secure, efficient, and trustworthy data ecosystem. The question isn’t whether privacy laws will impact your business—it’s whether you’re prepared to turn compliance into a strategic advantage.
Steps Companies Can Take to Strengthen Data Strategy
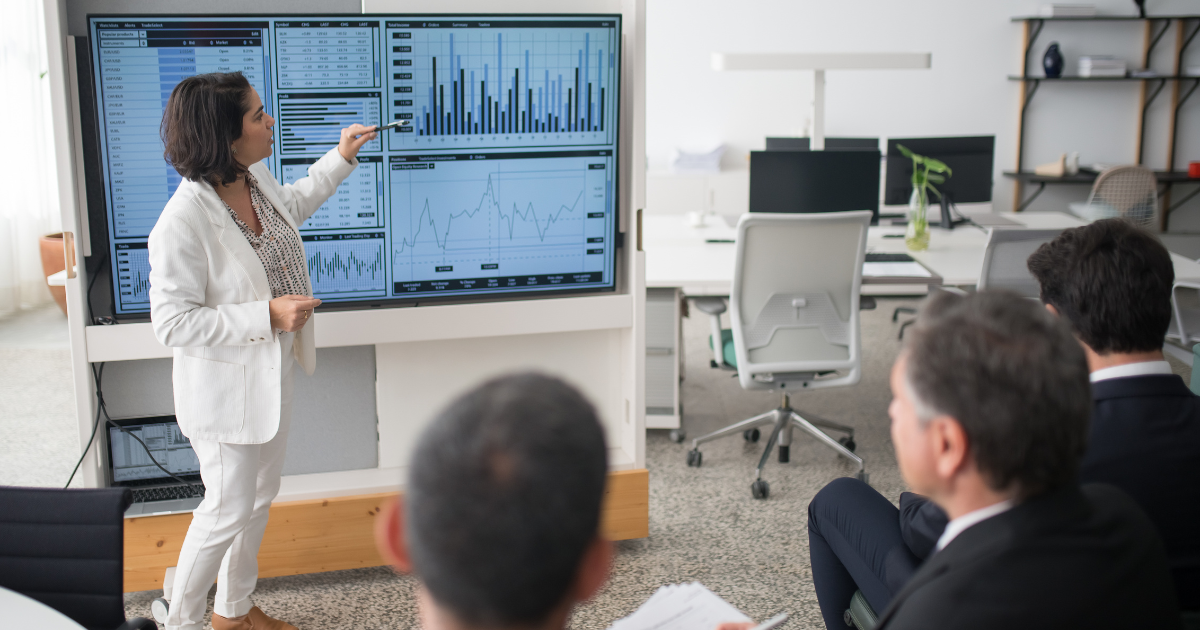
Conducting a Data Maturity Assessment
Before improving data strategy, companies need to understand where they currently stand. A data maturity assessment helps organizations identify gaps, set priorities, and create a roadmap for improvement.
Here’s how businesses can assess their data maturity:
- Evaluate Current Practices – Identify how data is collected, stored, and managed across different teams. Are processes standardized, or is data handling inconsistent?
- Use a Simple Maturity Scale – Companies can rate themselves on a 1-5 scale, where:
- Data is managed at a project level.
- Managed within specific departments.
- Governance exists across the organization.
- AI and advanced analytics are integrated.
- Data strategy is fully optimized and automated.
- Leverage Proven Frameworks – Instead of starting from scratch, businesses can use existing governance frameworks (like DAMA-DMBOK) to establish best practices quickly.
- Identify Quick Wins – If a company scores low on governance or quality, it should start with foundational improvements, such as creating data ownership roles and enforcing security policies.
Baseline assessments prevent costly mistakes when scaling AI, ensuring the organization is building on a solid foundation rather than patching issues later.
Implementing a Scalable Governance Framework
A strong governance model ensures data is accurate, secure, and accessible across the organization. However, many companies struggle with governance because they see it as restrictive rather than enabling.
To create an effective governance framework, companies should:
- Assign Clear Roles and Responsibilities – Data governance isn’t just IT’s job. Successful organizations have:
- Chief Data Officers (CDOs) will set the vision.
- Data Stewards to manage data accuracy and compliance.
- Governance Committees to align strategy with business objectives.
- Break Down Silos – Governance should connect business units, IT, and leadership, ensuring everyone follows the same policies.
- Use Automation for Compliance – AI-driven governance tools can help companies manage privacy laws, monitor data usage, and enforce access controls without slowing down operations.
A scalable governance framework balances structure and flexibility, allowing companies to innovate while staying compliant.
Aligning Data Strategy with AI and Future Innovations
Many companies rush into AI without fixing their data foundations first. Greg emphasizes that AI adoption should follow governance, not the other way around. If data is inconsistent, unstructured, or poorly managed, AI will produce unreliable insights, leading to costly failures.
Here’s how businesses can future-proof their data strategy:
- Treat AI as an Extension of Governance – Before deploying AI, organizations must ensure the following:
- Data is clean, structured, and secure.
- Employees understand AI risks and ethical considerations.
- Models are regularly monitored for bias and errors.
- Balance Innovation with Responsibility – Companies should experiment with AI while maintaining oversight. This means testing small use cases first and ensuring AI aligns with real business needs.
- Prepare for Future Regulations – Privacy laws are evolving fast. Organizations that embed compliance into their data strategy now will avoid expensive legal challenges later.
A well-structured data strategy doesn’t just support current operations—it positions companies to adapt, scale, and lead in AI-driven innovation.
Conclusion: Data Governance as the Foundation for AI-Driven Success
In today’s data-driven world, having data isn’t enough—turning it into a strategic asset is what sets businesses apart. Greg Hahn’s insights reveal a clear pattern: Companies eager to embrace AI and analytics often overlook the foundational elements that make those technologies effective. Without strong governance, data becomes a liability instead of a competitive advantage.
The takeaway? Slow down to speed up. Before scaling AI, companies must invest in governance, data quality, and privacy compliance. A well-structured data strategy doesn’t just protect businesses from risks—it unlocks smarter decision-making, operational efficiency, and future innovation.
So, where does your company stand? Assess your data maturity, implement governance as a business-wide initiative, and align AI with real objectives. The organizations that get this right won’t just keep up—they’ll lead the future of AI-driven decision-making.