AI is transforming industries, from finance and healthcare to hiring and customer service. But with great power comes great responsibility. What happens when AI makes a wrong decision—denying a loan unfairly, favoring certain job applicants, or generating misleading responses?
That’s where AI governance comes in.
Think of it as quality control for AI—ensuring that models are ethical, fair, transparent, and aligned with business goals. Without it, companies risk financial losses, legal trouble, and reputational damage.
Jim Olsen, CTO at ModelOp, sees this challenge firsthand. Many businesses focus on building AI models but forget about what happens after deployment. Over time, AI can drift, making worse decisions than when it was first trained.
So read on because we’re diving into what AI governance really is, why it’s crucial, and how businesses can implement it effectively.
Understanding AI Governance
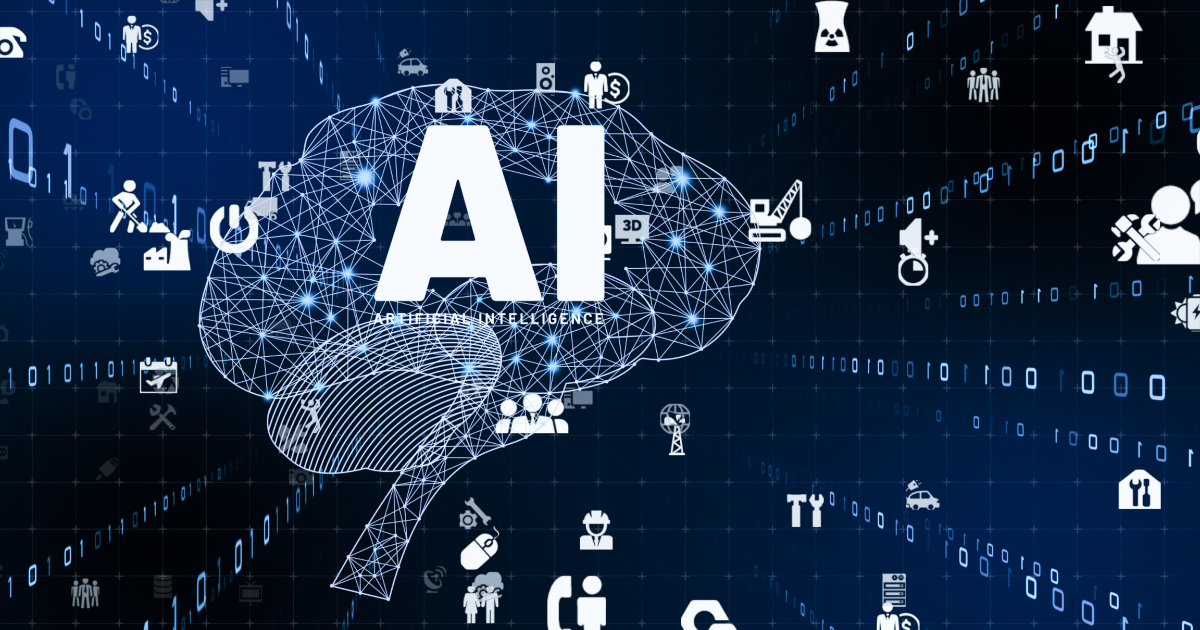
What AI Governance Means and Why It’s Essential
AI governance isn’t just about rules and compliance—it’s about knowing what your AI is doing and making sure it’s doing it right. It involves:
- Tracking AI decisions—so businesses can explain how and why AI makes choices.
- Preventing bias—to ensure fairness in AI-driven hiring, lending, or customer service.
- Ongoing monitoring—because AI performance changes over time and needs updates.
Jim puts it simply: AI doesn’t just need to work—it needs to work the right way, for the long run.
Without proper governance, businesses could end up with flawed models making critical decisions, which could lead to regulatory fines or discriminatory outcomes—even if they didn’t intend for that to happen.
How AI Governance Differs from Traditional Data Governance
Many companies assume that if their data is secure and compliant, their AI is, too. That’s not the case.
- Data governance focuses on privacy, security, and compliance—making sure data is protected.
- AI governance is about how AI uses that data—ensuring fairness, transparency, and accountability.
Example:
A bank might store customer data securely, following privacy laws.
But its AI-driven lending model could still deny loans unfairly based on patterns in historical data.
That’s why AI governance goes beyond data governance—it makes sure AI-powered decisions are responsible and justifiable.
The Core Pillars of AI Governance
To govern AI effectively, businesses need to focus on three major areas:
Model Inventory and Tracking
“You can’t govern what you don’t know exists.” – Jim Olsen
Businesses need a clear record of where AI is used, what it does, and how it impacts decisions.
- Where is AI deployed in the company?
- What decisions is it making?
- Are those decisions transparent and explainable?
Without proper tracking, businesses lose control over their AI, making it impossible to assess risk, comply with new regulations, or correct mistakes before they escalate.
Compliance and Risk Management
AI regulations are increasing worldwide—GDPR, the EU AI Act, U.S. state laws, and more.
Companies that fail to align AI with these laws risk:
- Hefty fines
- Legal action
- Damaged reputation
For example, the EU AI Act requires businesses to explain AI-driven decisions that affect consumers. A bank can’t just deny a loan—it must show why the AI made that decision.
If companies aren’t governing AI, they aren’t just risking compliance issues—they’re risking trust.
Ongoing Monitoring and Performance Checks
AI isn’t a one-and-done tool. Models can degrade over time, leading to bad predictions and biased outcomes.
Example:
A customer service chatbot trained on outdated data might start giving misleading financial advice.
A hiring model could unknowingly begin favoring certain demographics.
Jim highlights that AI needs continuous checks to detect these shifts before they become problems. The goal? Keep AI aligned with business objectives while ensuring fairness, accuracy, and reliability.
Measuring AI Performance Against Business Outcomes
AI governance isn’t just about following rules—it’s about ensuring AI delivers real value. A model might be 99% accurate, but if it doesn’t contribute to business growth, does it matter?
Jim Olsen highlights that businesses often focus too much on technical accuracy and not enough on business impact. AI models should be evaluated based on how they improve customer experience, efficiency, and revenue—not just precision metrics.
Key ways to measure AI performance:
- Customer impact – Does AI improve user experience, reduce wait times, or personalize services effectively?
- Operational efficiency – Is AI streamlining workflows, reducing costs, or making internal processes faster?
- Financial performance – Does AI contribute to higher sales, better fraud detection, or improved decision-making?
Example: A financial institution implementing AI for fraud detection should measure how many fraudulent transactions are prevented and how much money is saved, not just whether the AI’s prediction accuracy is high.
Overcoming AI Governance Challenges and Making It Work
Even though AI governance is crucial, many businesses struggle with it. Jim highlights the most common challenges:
No Universal AI Regulations
Unlike industries like finance or healthcare, AI governance doesn’t have a universal rulebook.
Example:
A company using AI in hiring, lending, and fraud detection must follow different standards for each case. The EU has strict laws, while U.S. regulations vary by state.
This makes compliance feel like a moving target, leaving businesses confused about where to focus.
Balancing Compliance and Innovation
Governance adds control, but AI thrives on rapid experimentation. Businesses often feel stuck between:
- Moving fast and risking mistakes, or
- Being too cautious and slowing down innovation.
Jim compares this to early software development, before DevOps introduced structured processes. AI needs a balance—structured governance without killing innovation.
AI Bias and Ethical Risks
AI models are trained on real-world data, which means they inherit real-world biases.
Example:
- A hiring model trained on past applications might favor certain demographics without meaning to.
- Without governance, businesses won’t even realize AI is reinforcing discrimination until it’s too late.
The solution? Regular bias audits and fairness testing.
AI Model Drift
AI doesn’t stay accurate forever. Over time, models begin making less reliable decisions.
- A financial forecasting AI might misinterpret economic trends.
- A chatbot might generate misleading answers.
Without governance, businesses won’t realize their AI is broken until customers complain—or regulators step in.
Proving AI Decisions Are Trustworthy
One of the biggest hurdles? AI explainability.
When AI rejects a loan application, flags a fraudulent transaction, or makes a medical recommendation, businesses need to prove why.
But many AI models operate as black boxes, making it hard to trace decisions.
Jim highlights that companies must invest in transparency because regulators, customers, and executives all demand clear, understandable AI outcomes.
How Can Engineering Strategy Contribute to Fair and Reliable AI Governance?
Frontline leadership engineering insights are crucial in shaping AI governance frameworks that prioritize fairness and reliability. By integrating diverse perspectives and ethical considerations into engineering practices, organizations can develop AI systems that align with societal values, thereby fostering trust and accountability in their deployment and operation.
How Businesses Can Make AI Governance Work
AI governance isn’t just about ticking compliance boxes—it’s about keeping AI reliable, fair, and transparent. Many companies rush to deploy AI but don’t track its long-term impact. Over time, models can drift, become biased, or make unreliable decisions.
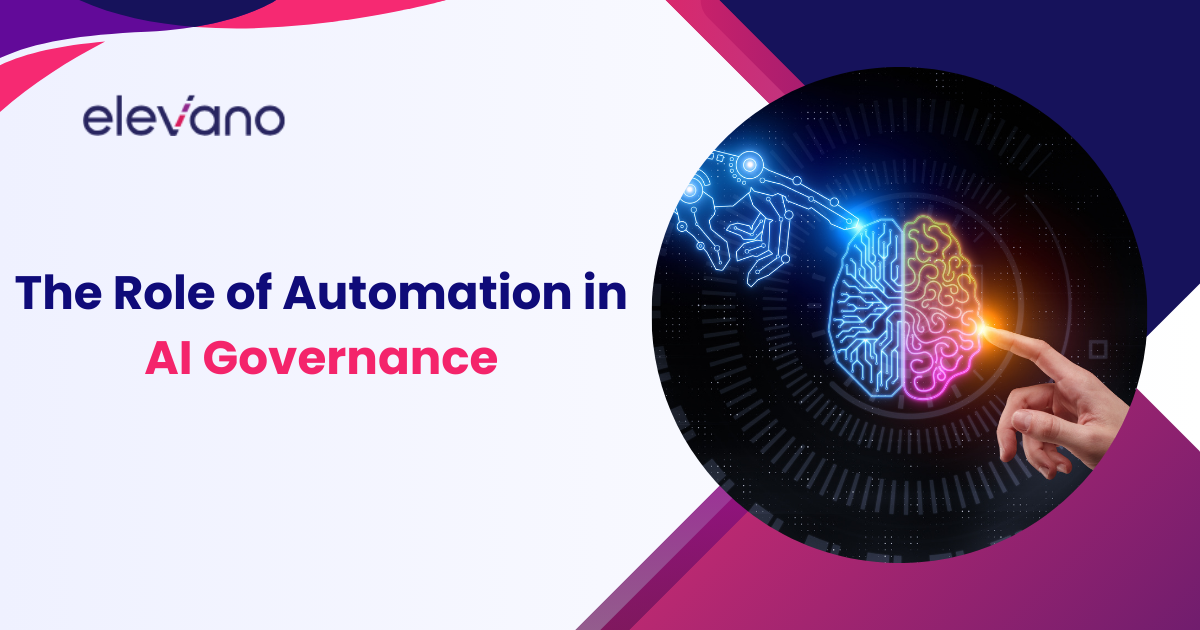
Jim Olsen breaks down how businesses can govern AI without slowing innovation or adding unnecessary red tape.
The Role of Automation in AI Governance
Governance doesn’t have to slow AI adoption. Automation can make oversight easier, reducing errors and ensuring compliance without constant manual checks.
Jim Olsen highlights a key issue: AI generates too much data for businesses to track manually. Instead, companies should use AI-powered governance tools that:
- Detect bias before deployment – AI can flag patterns of discrimination early.
- Monitor AI performance in real-time – Dashboards can catch AI drift and anomalies.
- Automate compliance – AI can scan for regulatory updates and ensure models meet new standards.
Example: A hiring AI can have built-in bias detection, automatically flagging patterns where certain demographics are favored or excluded.
Now, here comes the good part—automation doesn’t just reduce error; it makes AI governance scalable. Instead of burdening teams with manual compliance checks, businesses can focus on improving AI models while ensuring they remain fair, reliable, and compliant.
Track Every AI Model
If you don’t know where AI is being used, you can’t govern it. Businesses need a clear inventory of all AI models—what they do, where they’re deployed, and how they impact decisions.
Why it matters: If a new bias regulation is introduced, businesses need to update AI-driven hiring tools quickly. Without a centralized AI registry, compliance becomes chaos.
Action step: Maintain an AI inventory and review it regularly.
Set Practical Governance Policies
Not all AI needs the same level of oversight. A fraud detection AI in banking requires stricter governance than an AI-powered email assistant.
Key areas to cover:
- Bias detection – Prevent AI from making unfair decisions.
- Explainability – AI decisions shouldn’t be a “black box.”
- Compliance – Stay ahead of legal risks instead of scrambling to fix them later.
Pro Tip: AI governance should be flexible, ensuring safety without stifling innovation.
Monitor AI Continuously—Don’t “Set It and Forget It”
AI models change over time—and not always for the better. An outdated AI can start making inaccurate or biased decisions without anyone noticing.
Example:
- A chatbot trained on old financial data might start giving misleading investment advice.
- A fraud detection AI could fail to recognize new scam patterns.
How to fix it:
- Automated alerts when AI performance declines.
- Regular audits to catch bias creep before it leads to compliance issues.
Keep Humans in the Loop
AI should support, not replace, human decision-making—especially in high-stakes situations.
Where human oversight is critical:
- Healthcare – AI-generated diagnoses should be reviewed by doctors.
- Hiring – Recruiters must double-check AI-filtered candidates.
- Financial lending – AI shouldn’t auto-reject loan applications without human validation.
Best practice: Define clear policies on when AI decisions need human review. AI should assist, not make the final call in life-altering decisions.
Jim Olsen makes one thing clear: “AI needs oversight—not just at launch, but for its entire lifecycle.”
Conclusion: Making AI Governance Work for the Future
AI is no longer just a tool—it’s shaping decisions that impact businesses, customers, and entire industries. But without the right governance, even the most advanced AI can become a liability. Jim Olsen’s insights make one thing clear: AI governance isn’t just about avoiding mistakes—it’s about making AI work better, safer, and smarter.
Companies that take governance seriously will stay ahead of regulations, reduce risks, and build trust with customers. Those that ignore it? They’ll face compliance headaches, biased outcomes, and AI models that become more of a problem than a solution.
So what’s next? Businesses need to track their AI, monitor performance, automate governance where possible, and always keep human oversight in critical decisions. AI is a powerful tool, but only when it’s transparent, explainable, and aligned with business goals.
Now here comes the good part—the companies that invest in AI governance today won’t just stay compliant; they’ll lead the future of responsible AI.